Enhancing Scientific Discoveries with Artificial Intelligence
EMSL’s Integration 2022 explores the use of machine learning and AI for science

Artificial intelligence and machine learning can enhance data analysis of complex results for mass spectrometry. The EMSL Integration Meeting 2022 will feature a session on automated discovery of molecular profiles across omics as it pertains to mass spectrometry. (Photo by Andrea Starr | Pacific Northwest National Laboratory)
Scientists produce a lot of data in their experiments, from genome sequences and protein structures to elemental analysis and microscope images. It’s more data than scientists, themselves, have time or the ability to comb through.
Computer-based techniques, such as artificial intelligence (AI) and machine learning (ML), can help researchers use this data in meaningful ways. AI allows computers to mimic human logic and reasoning. ML is a subset of AI that includes statistical analysis to improve tasks. Together, these techniques help scientists mine through massive amounts of data much quicker than a human could alone.
The upcoming EMSL Integration Meeting 2022 hosted by the Environmental Molecular Sciences Laboratory (EMSL), a Department of Energy, Office of Science user facility, will explore the use of such techniques for biological and environmental science. The meeting, which takes place on October 4–6, 2022, will feature plenary speakers, poster sessions, and live demonstrations of AI and ML applications for systems and synthetic biology, imaging, and mass spectrometry.
Artificial intelligence, machine learning, and efficient experimentation
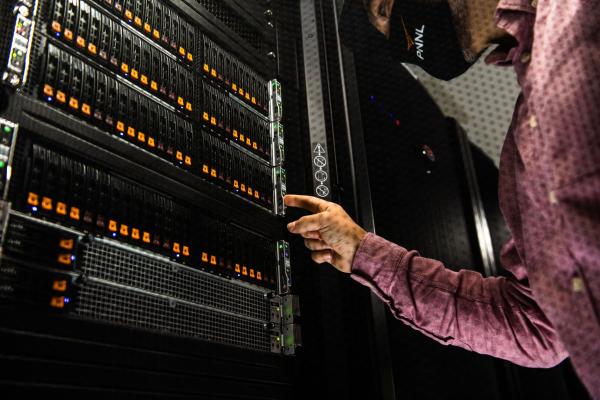
In systems biology, researchers investigate the complexities of a cell or organism, from molecular-level interactions to collective behaviors. In synthetic biology, scientists attempt to design biological systems for specific applications. AI and ML help bridge the gap between the two disciplines so that they may inform each other.
Researchers like Miguel Fuentes-Cabrera from Oak Ridge National Laboratory and Arvind Ramanathan from Argonne National Laboratory use data from systems biology to guide the design of new biological systems. Both researchers will be featured as plenary speakers at the upcoming EMSL Integration Meeting 2022.
Fuentes-Cabrera uses AI and a simulation technique called agent-based modeling to study microbial populations. Information from this type of modeling can then be used to design synthetic populations of microbes.
“With AI and agent-based modeling, we might be able to make our experiments more autonomous,” said Fuentes-Cabrera. “Instead of a trial-and-error process for every change we want to introduce in a microbial population, we can simulate what the outcome of that change would be and proceed with only the most successful changes.”
Ramanathan uses AI and ML to mine protein and genome sequence data to reverse engineer reaction pathways of interest. The computer programs search through the data to find sequences that may grant a certain function.
“In a cell, reactions are not done in isolation,” said Ramanathan. “Many enzymes may be involved in the pathway to make the reaction proceed. AI and ML can identify different enzymes and potential new pathways to complete the reaction.”
AI and ML are useful in other biological and environmental research areas, as well. Techniques such as cryo-electron microscopy (cryo-EM) produce high-resolution images of large biological molecules and cellular components. However, the images can often be very complex to analyze. AI helps process these images to reconstruct three-dimensional structures of molecules.
Mass spectrometry is a powerful technique for investigating different molecules and complexes based on their mass-to-charge ratio. Technological advancements in the field have made mass spectrometry especially sensitive for a wide variety of different applications like detecting contaminants, such as pesticides in soil samples, or characterizing proteins in molecular complexes. Like with other techniques, AI and ML can enhance data analysis of complex results for mass spectrometry.
These applications of AI and ML for biological and environmental science and more will be discussed at the upcoming EMSL Integration Meeting 2022. Plenary speakers include Fuentes-Cabrera and Ramanathan, as well as Olga Vitek from Northeastern University and Jake Beal from Raytheon BBN Technologies, among others.
Researchers interested in attending the meeting should register on the meeting website.